Common Data Collection Mistakes and How to Avoid Them
- Fadl Rahman Essel
- Jan 24
- 5 min read
Updated: 9 hours ago
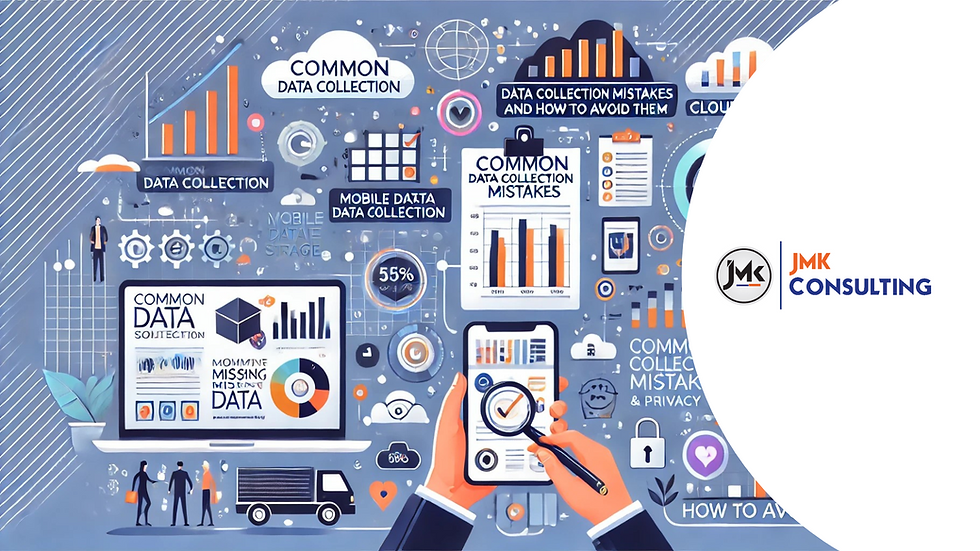
Collecting accurate and reliable data is more important than ever in today's data-driven world. However, common data collection mistakes can lead to flawed insights, poor decision-making, and missed opportunities across business, healthcare, and research industries. Despite its importance, the data collection process is often riddled with challenges that can undermine the integrity of the information gathered.
"Poor data quality costs businesses 20-35% of their operating revenue, making it essential to invest in robust data collection strategies." — Thomas C. Redman, Data Quality Expert and Author of Data Driven
In this article, we will explore frequent pitfalls in data collection and provide actionable strategies to help you avoid them, ensuring your data remains accurate, relevant, and valuable.
1. Undefined Objectives
Mistake: Initiating data collection without clear, specific objectives can lead to gathering irrelevant or excessive data, making analysis cumbersome and less effective.
Example: A marketing team collects data on all customer interactions without focusing on specific metrics like customer satisfaction or purchasing behaviour, resulting in an overwhelming amount of data with no clear insights.
Solution: Clearly define the purpose of data collection by setting specific, measurable objectives. Determine what information is essential to achieve your goals and focus on collecting that data. This targeted approach streamlines the process and enhances the relevance of the data collected.
Learn more about common pitfalls in research to avoid such issues.
2. Inadequate Planning and Preparation
Mistake: Failing to plan the data collection process thoroughly can result in incomplete or biased data, as well as logistical challenges during collection.
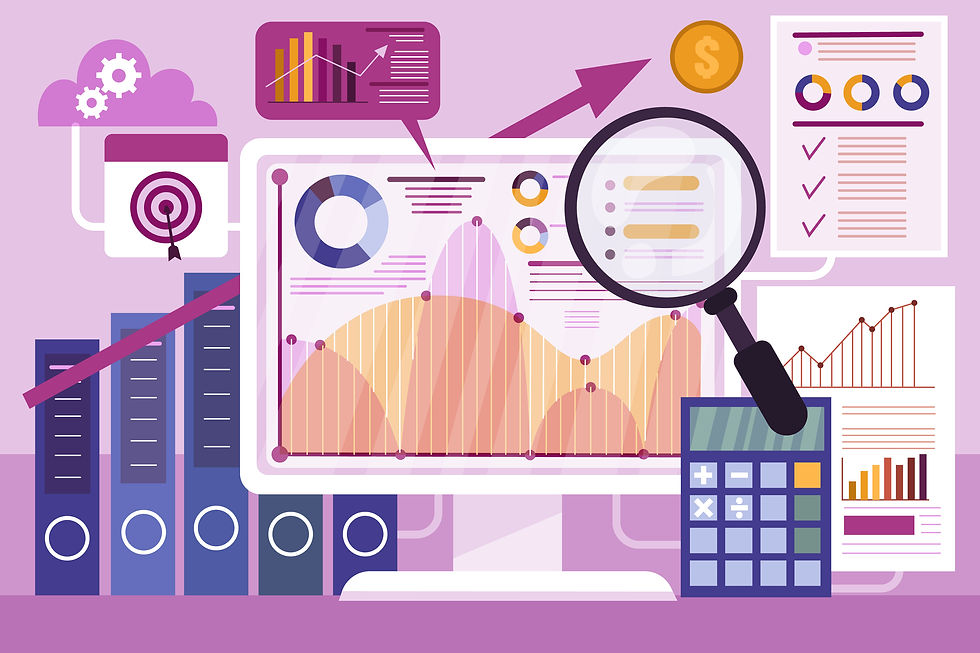
Example: A research team starts a survey without determining the sample size or demographic representation, leading to skewed results that don't accurately reflect the target population.
Solution: Develop a comprehensive data collection plan that includes defining the target population, selecting appropriate data collection methods, and establishing protocols to ensure consistency. Proper planning helps in anticipating potential challenges and addressing them proactively.
Read more about best practices in data collection to avoid costly surprises.
3. Poorly Designed Data Collection Instruments
Mistake: Using data collection instruments with ambiguous, leading, or complex questions can result in inaccurate or unreliable data.
Example: A survey includes double-barreled questions like, "How satisfied are you with our product quality and customer service?" making it unclear which aspect the respondent is addressing.
Solution: Design clear, concise, and unbiased questions. Pilot testing the instruments on a small group can help identify and rectify issues before full-scale deployment, ensuring the questions elicit the intended information.
Learn how to design better surveys effectively.
"Good data is not collected by chance; it is the result of careful planning and execution." — Dr. Jane Murphy, Professor of Data Science, Harvard University
4. Inadequate Training of Data Collectors
Mistake: Data collectors who are not properly trained may introduce inconsistencies, biases, or errors in the data collection process.
Example: In a field study, data collectors interpret survey questions differently, leading to variations in how responses are recorded.
Solution: Provide comprehensive training to all individuals involved in data collection. This training should cover the objectives of the study, detailed procedures, ethical considerations, and methods to handle unexpected situations, ensuring uniformity and reliability in data collection.
Discover strategies to avoid data collection mistakes and ensure consistency.
5. Sampling Errors
Mistake: Errors in selecting the sample, such as using a non-representative sample or having a biased sampling method, can compromise the validity of the data.
Example: Conducting an online survey about internet usage habits but only distributing it through social media platforms, thereby excluding individuals who do not use social media.
Solution: Employ appropriate sampling techniques to ensure the sample accurately represents the target population. Random sampling methods can help minimize biases and enhance the generalizability of the findings.
Learn more about avoiding sampling errors to improve data reliability.
6. Data Entry Errors
Mistake: Manual data entry is prone to errors such as typos, omissions, or incorrect data formatting, leading to inaccurate datasets.
Example: In a healthcare study, patient ages are entered manually, and due to typographical errors, some ages are recorded incorrectly, affecting the study's outcomes.
"Human error in data entry can cost organizations significant time and resources. Investing in automation and validation processes is key to ensuring data accuracy." — Susan Walsh, The Classification Guru and Data Quality Expert
Solution: Implement data validation checks and, where possible, automate the data entry process using digital tools to reduce human error. Regular audits of the data can also help identify and correct errors promptly.
Read about Data Quality: 10 mistakes not to make for better accuracy.
7. Ignoring Data Privacy and Ethical Considerations
Mistake: Failing to adhere to data privacy laws and ethical standards can lead to legal repercussions and loss of trust among participants.
Example: Collecting personal data without informed consent or not securing the data properly, resulting in unauthorized access.
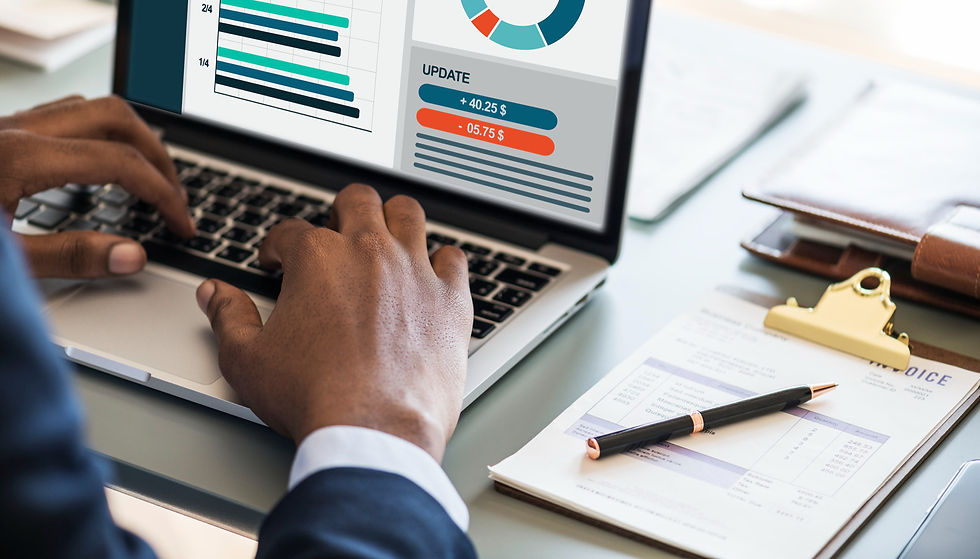
Solution: Familiarize yourself with relevant data protection regulations, such as GDPR or HIPAA, and ensure compliance throughout the data collection process. Obtain informed consent from participants and implement robust data security measures to protect sensitive information.
Link to embed: What Is Data Collection: Methods, Types, Errors
8. Overlooking Data Cleaning and Validation
Mistake: Neglecting to clean and validate data can result in datasets containing duplicates, inconsistencies, or outliers that can skew analysis results.
Example: A dataset includes multiple entries for the same individual due to variations in name spelling, leading to inaccurate counts and analysis.
Solution: Perform thorough data cleaning to identify and rectify errors, inconsistencies, and duplicates. Utilize data validation techniques to ensure the accuracy and integrity of the dataset before proceeding to analysis.
Link to embed: How to avoid 10 common mistakes in data reporting
9. Inadequate Documentation
Mistake: Failing to document the data collection process, including methodologies and any issues encountered, can hinder reproducibility and transparency.
Example: A research project lacks detailed records of how data was collected and processed, making it difficult for other researchers to replicate the study or understand the context of the findings.
Solution: Maintain comprehensive documentation of the data collection process, including methodologies, tools used, data sources, and any challenges faced. This practice enhances transparency and facilitates future research or audits.
Link to embed: Common Pitfalls In The Research Process
10. Not Pretesting Data Collection Instruments
Mistake: Deploying data collection instruments without pretesting can result in unforeseen issues that compromise data quality.
Example: A survey is distributed without pretesting, and respondents find certain questions confusing, leading to incomplete or inaccurate responses.
Solution: Conduct a pilot test of your data collection instruments with a small subset of your target population. This testing helps identify and address potential problems, ensuring the tools function as intended in the actual study.
Link to embed: 8 Common Errors In Data Collection And How To Resolve Them
Conclusion
Avoiding common data collection mistakes is essential to ensuring the accuracy, reliability, and usefulness of your data. By defining clear objectives, planning thoroughly, using well-designed instruments, and adhering to ethical standards, you can enhance the quality of the data you collect. Additionally, leveraging automation tools, conducting regular audits, and continuously refining your methods will help you achieve better results over time.
In today’s rapidly evolving digital landscape, organizations that prioritize high-quality data collection practices are better positioned to make informed decisions, drive innovation, and maintain compliance with data regulations. Whether you're conducting market research, academic studies, or business analytics, following best practices in data collection is crucial for achieving meaningful insights and long-term success.
Let us know your thoughts in the comment box below!
Additional Resources on Common Data Collection Mistakes
For further reading and to improve your data collection strategies, consider exploring these resources:
By incorporating these best practices and avoiding the mistakes discussed in this article, you'll be on your way to making data-driven decisions with confidence in 2025 and beyond.
Comments